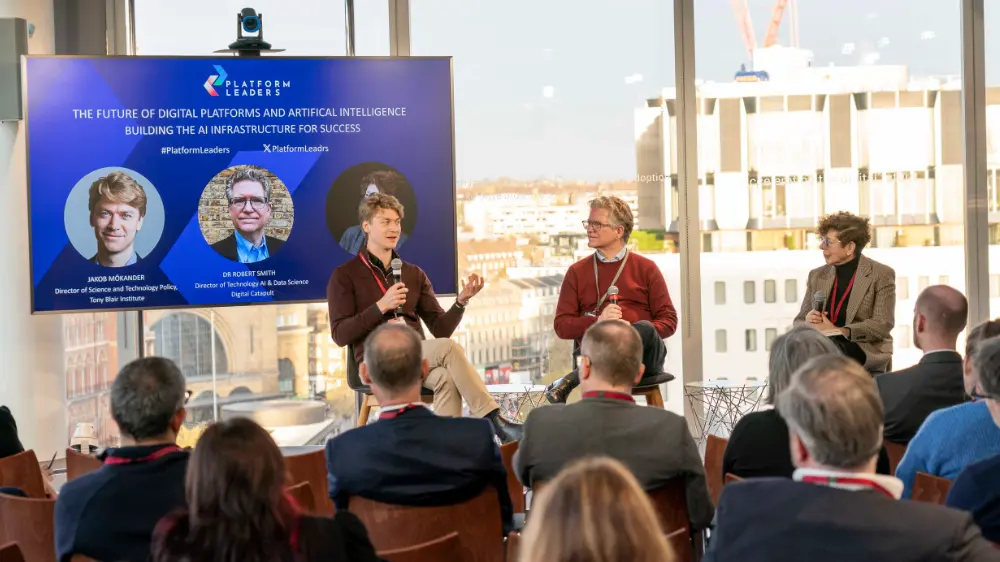
Building the AI Infrastructure for Success
November 25, 2024
AI is no longer just an experiment – it’s revolutionising industries and transforming how businesses operate. Organisations are racing to build powerful infrastructures that unlock the full potential of advanced AI models, driven by massive investments from cloud providers. These scalable solutions promise to seamlessly embed AI into workflows, setting the stage for unprecedented innovation and growth.
Still, the dramatic technological progress that AI is driving comes with its challenges, and this is especially the case for firms that are making key decisions about how to leverage AI within their business. “If AI is going to be the key to everything – and I think everyone agrees it will be – then what needs to be kept in-house?” asks Jennifer Schenker, Founder & Editor-in-Chief of The Innovator.
From stacks to chains: A new perspective on AI architecture
To begin with, firms need to understand the operational framework required for AI. Robert Smith, Director of Technology AI and Data Science at Digital Catapult, and author of the acclaimed book Rage Inside The Machine, thinks of AI infrastructure in different terms from a firm’s typical technological toolkit. “I wouldn’t call it a stack, I call it a chain,” he says. This chain starts from foundational elements like data storage and processing (GPUs, TPUs), then progresses to data handling frameworks, AI frameworks and finally, to MLOps (Machine Learning Operations).
“Now, what’s going on in AI today is that a lot of the AI framework pieces have been commoditised,” says Robert, referring to frameworks provided by major players like Microsoft, Amazon and Oracle. “They own the space in many ways,” he adds. Although these players dominate at the framework level, MLOps is an area of active innovation for SMEs and corporate firms alike. This includes tools for monitoring and retraining models to address issues like data staleness or biases. “These are tools that you didn’t need in the past in the DevOps world that you do need now in the MLOps world,” explains Robert.
According to Robert, MLOps is still an evolving field with significant opportunities for new providers to shape the landscape. Unlike the more mature layers of the AI infrastructure chain, Robert says that the key providers in MLOps are not yet firmly established: “I think that’s a moving target.”
The role of government in AI development
The role that regulators will play in allowing AI to operate at scale is another moving target, which Jakob Mökander knows firsthand. As Director of Science & Technology Policy at the Tony Blair Institute, he consults with governments around the world on fostering innovation ecosystems for digital technologies and AI. He’s familiar with the challenges these governments face, including regulatory fragmentation, balancing digital sovereignty with the need for efficiency and navigating geopolitical dynamics. Decisions like whether to digitise critical systems such as healthcare or national security are made more complex amidst these competing concerns. “Many of them are hesitating because [finding] the right layers of protection between national and digital sovereignty […] is hard to do in practice,” explains Jakob.
There are also ongoing global disparities in compute power and infrastructure. When it comes to data centre investment, Jakob says that one country is far outpacing most of the world:
“[In terms of AI Infrastructure,] If you exclude China, the US is out-investing the rest of the world combined”
Jakob Mökander, Director of Science & Technology Policy, Tony Blair Institute
Export controls and a concentration of advanced chip production in controlled geographies exacerbate these inequalities, making access to essential technologies harder for some regions.
To address these issues, Jakob advocates for policies that promote platform interoperability, talent mobility and shared regional compute clusters. However, he notes that conflicts in regions with limited compute access add another layer of difficulty to creating these shared resources: “In order to make the innovation ecosystem flourish, we need to not just think of the technical stack, we also need to think about the geopolitical considerations around it.” In Jakob’s opinion, governments need to focus on investing in generalisable parts of the AI chain and provide a stable foundation that innovative entrepreneurs and developers can build on.
Navigating AI’s risks and opportunities
The emerging dominance of a few major players in AI infrastructure could have significant competition implications. The UK Competition and Markets Authority has already raised concerns about this concentration of power, and Robert suggests that governments may need to address it through antitrust measures.
Beyond competition, there are also cultural risks. “The dominance of a few players means the dominance of fewer cultures,” Robert notes. However, if utilised well, large language models (LLMs) could also prove to be a powerful defensive tool for endangered minority languages. “It could be that we have an opportunity to preserve the knowledge of cultures that might be lost if we don’t allow the dominance of just a few cultures over the infrastructure,” he says.
Jakob also identifies the legal and ethical questions that AI raises, pointing to how Google’s new AI summaries differ from traditional search results. He illustrates: “If I ask Google ‘who won the gold medal in rowing in 1994’, I will get an answer because there is consensus among the sources. But if I ask ‘was the Russian meddling in the 2020 election significant’, there will not be [consensus].” This introduces an editorial function that AI fulfils, particularly difficult for contentious topics. Robert agrees that more attention is needed in this area: “When I read Google’s AI summaries, those biases are an emergent phenomenon for the most part,” he says. “They’re not Google’s biases, they’re biases that are so subtly embedded in complex, massive training data that they’re not only intractable to me, they’re intractable probably to everyone, and this is a real concern.”
The need for a nuanced approach to AI regulation is another challenge. In Jakob’s view, the current discussion on AI regulation would be similar to designing regulations for “vehicles” without distinguishing between bicycles, cars or spaceships – an analogy also referenced in the recent book “AI Snake Oil: What Artificial Intelligence Can Do, What It Can’t, and How to Tell the Difference” by Arvind Narayanan and Sayash Kapoor.
Indeed, AI governance spans diverse areas like competition law, product safety and national security, each requiring tailored frameworks. “All of these things are important – competition law, product law, national security – but it might not necessarily be helpful to collapse them all into one and call it AI regulation,” says Jakob. “That is the problem right now when we’re talking about AI regulation and governance: we’re talking about vehicles when we need to be talking about spaceships, cars and bikes in different contexts.”
For Jakob, there is a balance between harnessing the opportunities of AI and addressing its risks. “There is no use in looking away from the incredible benefits that can be had in particular sectors when the [AI models] are well designed.” However, he continues, realising these benefits requires robust policies in areas like national security, data privacy, human rights and anti-discrimination. In his opinion, these policies serve as essential enablers alongside technical resources like data and compute.
Customising AI solutions: Local vs. global approaches
Undeniably, data and compute are vital enablers, however. The full power of LLMs arises from a combination of advanced architecture, substantial computational resources and access to vast amounts of data. As Robert describes it, while this combination has driven impressive breakthroughs, it has a significant limitation: in specialised fields like aerospace engineering, much of the valuable data remains locked in proprietary or sovereign silos. If industries could access and share these datasets, it could enable the creation of highly advanced, domain-specific AI models capable of transformative applications.
“There’s a huge amount of work to be done between companies and nations about how to advance the power of AI through data sharing”
Robert Smith, Director of Technology AI and Data Science, Digital Catapult
Robert emphasises the importance of developing technologies and frameworks that facilitate secure and effective data sharing, a critical step for unlocking AI’s full potential in specialised domains.
Resource limitations that can hamper innovation within a given industry are also challenges for national governments, according to Jakob. “Very few countries on this earth can afford to build the full stack internally, and that would also not be good. That would actually push us further down the line of geopolitical fragmentation,” he thinks. Instead, he says that governments should segment their AI use cases, evaluate associated risks and adopt technologies tailored to their local context. For example, while not every country needs to develop its own large language model, fine-tuning models on local data can yield more effective results. “It would be a huge mistake to take healthcare algorithms trained in Europe or North America and apply them in Sub-Saharan Africa or in the Middle East,” thinks Jakob. In particular some melanoma cancer detection AI algorithms, trained on lighter skin, have been shown to give inaccurate results for darker skin patients. “There might be cases where you want to make some investment in local domestic infrastructure for the most critical cases.” To balance integration, sovereignty and efficiency, he believes that countries are not faced with a binary choice between local and global sourcing. Rather, he says: “There will be a plurality of approaches needed for most countries.”
Defining open source in the AI age
One potential component of any approach could be open source AI, as Jennifer notes.
“[Open source] is a way that companies can perhaps not be tied completely to the large end providers from the United States, but also help them potentially meet cost and sustainability goals by allowing them the freedom to use small language models when LLMs are not necessary”
Jennifer Schenker, Founder & Editor-in-Chief, The Innovator
The specifics of what open AI entails continue to evolve, however, with Robert pointing out the recent definition released by the Open Source Institute. It focuses on three primary aspects: open architecture, which involves transparency in how AI models are structured and built; open weights, referring to making the model’s parameters accessible; and open training data, the most controversial aspect. “AI, as it’s defined now, is critically important to the advancement of AI for lots of objectives: for fairness, sustainability, ubiquitous use, all those kinds of things,” says Robert.
While the Open Source Institute suggests that an open AI system should by definition be reproducible, in Robert’s opinion, it sidesteps a firm stance on whether training data has to be entirely open. Emerging technologies are aimed at ensuring data sovereignty – allowing individuals or companies to share data while protecting sensitive or proprietary information – but these are still in development. And although AI tools like MidJourney may have explicit restrictions against using proprietary data, these restrictions can be bypassed if requests are rephrased. “If you ask it to draw Mario, it will tell you it can’t. It’s a copyrighted character,” explains Robert. “If you ask it to draw a cartoon plumber, it draws Mario reliably. And this is true across all the AI models.” Similarly, language models can inadvertently replicate copyrighted material like New York Times articles. “This data issue is going to continue to nag us,” Robert predicts. “It is a key challenge within generative AI today that we have not yet solved and is a key research issue. It’s also a key issue about investment and hesitancy to invest.”
To address concerns around data privacy and intellectual property laws, Jakob believes there is a clear need for thoughtful regulatory updates in the AI era. “When we talk about updating regulation in the age of AI, that can cut both ways. That doesn’t always mean stricter laws or upholding existing laws,” he explains. Digital ecosystems, with their unique characteristics like non-rival, shareable resources, may require a different approach to governance.
Integrating AI into business workflows
The question for many entrepreneurs remains: how can firms implement Generative AI today and start reaping its benefits? For Robert, the key to success lies in effectively balancing human and machine capabilities within workflows. “What we have now is a tool to help us distinguish between what a machine does best and what a human does best, and make those workflows work,” he says. “The companies that will succeed are the ones that make good decisions about that.”
Jakob adds a note of caution when considering the potential of AI in improving productivity. “Any of the statistics that are projections of the future should be taken with a big grain of salt. They’re probably all wrong, but they can give us a sense of proportion.” Even so, he notes that while generative AI may contribute to a productivity boost, this accounts for only 30% of the overall gains expected from automation and AI. The majority of efficiency improvements still lie in areas like machine learning, automated decision-making and process management.
Still, Jakob sees untapped potential in Generative AI. “To use [Gen AI] as a search engine or to generate ideas is perhaps a limited use case.” Instead, he prefers to use it collaboratively, leveraging AI to refine his own ideas and improve written communication for diverse audiences, such as journalists, political leaders or the public. This synergy between human creativity and AI capabilities represents an underutilised opportunity for firms willing to experiment.
Robert, meanwhile, emphasises the importance of careful adoption. “There are processes that run at a certain cadence, and when you convert them to automated cadences, they can run faster,” he says. “But what you discover is that the process automation may have overlooked criteria and steps that you didn’t realise you were actually doing implicitly in the slower model.” He stresses the importance of flexibility in workflows, noting that firms must address exception cases and adjust operations to fully integrate machine learning tools. “How do you have to adjust your operations to cope with the idea of [machine learning]?” he asks, framing this as a fundamental challenge for businesses.
AI: The question of sustainability
As AI adoption accelerates, its sustainability remains a critical yet often overlooked concern. LLMs, while transformative, are energy-intensive, contributing to significant environmental challenges. “There’s a lack of standardisation on the measurement and also on the responsibility to report,” noted Jennifer. This inconsistency further complicates efforts to evaluate and mitigate the environmental impacts. With corporations worldwide under mounting pressure to adopt greener practices, addressing this transparency gap is essential, says Jennifer: “We’ve got to get the balance right, and we’re almost out of time.”
Despite the challenges, Jakob offers a more optimistic perspective.
“Artificial intelligence will, in the long term, be a net positive for our ability to address climate change”
Jakob Mökander, Director of Science & Technology Policy, Tony Blair Institute
Although data centres already account for around 1% of national energy consumption in most industrialised countries – and even higher in Ireland and across Scandinavia – he argues that AI can still contribute to sustainability. By optimising processes, solving complex problems and reducing reliance on material resources, AI could become a valuable ally in addressing global environmental challenges.
Strategic takeaways for AI integration
When it comes to operationalising AI, Robert’s message is clear: “AI is bigger than just Generative AI.” While LLMs are transformative, domain-specific models could ultimately be more impactful, though their development is also more complex. He highlighted the critical importance of integrating AI into human workflows, focusing on the synergy between human and machine tasks. This integration represents both the most pressing research challenge and a key opportunity for creating value.
As the AI landscape evolves rapidly, Jakob thinks that adaptability in both strategic and regulatory decisions is key. “I would caution both companies and governments not to over-index on the current model,” he says. “This particular computational technique did not exist three or four years back, and in just three or four years we might have new kinds of computational techniques, whether they are hybrid, multimodal or non-symbolic systems.” Instead, he advises focusing on long-term investments in foundational layers of AI ecosystems to avoid technological lock-in. For governments, he advocates for developing dynamic, flexible regulations that align with the ongoing evolution of architectures and algorithms.
Overall, Jakob recommends focusing on real-world problems rather than prioritising AI itself. As AI becomes more integrated into various fields, he predicts it will lose its uniqueness as a selling point. Instead, Jakob says, the focus should shift to how AI improves specific processes, such as healthcare, drug discovery or democratic transparency. Success depends on accurately modelling real-world problems, validating AI outputs and ensuring effective feedback loops. The priority lies in problem-solving and improvement, ensuring that AI remains a tool to enhance human potential rather than an end in itself.
Join the conversation
This panel with Jakob Mökander, Jennifer Schenker and Robert Smith was part of the Platform Leaders event organised by Launchworks & Co on the 13th of November 2024 at Digital Catapult. Bringing together hundreds of experts from around the globe, both in person and online, Platform Leaders creates a unique space for entrepreneurs, academics, practitioners and policymakers to explore key issues and drive meaningful conversations. Browse the full agenda and list of speakers and catch up on videos and articles on the Platform Leaders website. And don’t miss the chance to dive deeper – join the community to stay informed about future events!
The Platform Leaders initiative has been launched by Launchworks & Co to help unlock the power of communities and networks for the benefit of all. All Launchworks & Co experts live and breathe digital platforms and digital ecosystems. Some of their insights have been captured in best-selling book Platform Strategy, available in English, French and Japanese.
The Platform Leaders initiative has been launched by Launchworks & Co to help unlock the power of communities and networks for the benefit of all. All Launchworks & Co experts live and breathe digital platforms and digital ecosystems. Some of their insights have been captured in best-selling book Platform Strategy, available in English, French and Japanese.